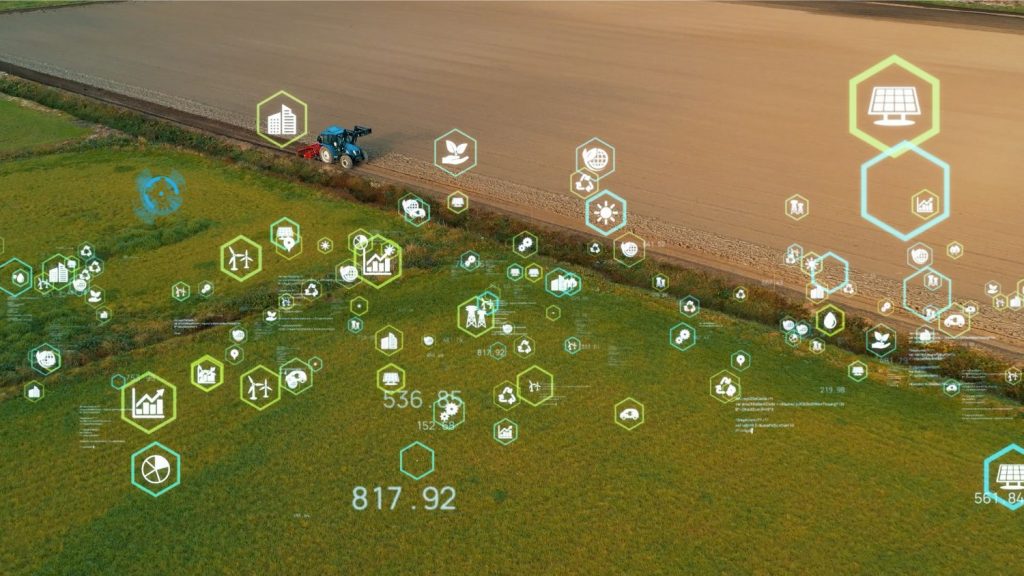
With various applications of AI in agriculture to reduce carbon emissions, the agricultural sector is becoming more sustainable. Agriculture is responsible for approximately 24% of global greenhouse gas emissions, primarily through deforestation, livestock production, and the use of synthetic fertilizers. However, advancements in artificial intelligence (AI) offer promising solutions to reduce carbon emissions and enhance sustainability in farming. In this article, we explore the key applications of AI in agriculture that contribute to lowering carbon footprints.
1. Precision Farming for Optimized Resource Use
AI-powered precision farming techniques enable farmers to use resources like water, fertilizers, and pesticides more efficiently. By employing machine learning algorithms and data analytics, AI can analyze various factors such as soil health, weather conditions, and crop requirements to provide precise recommendations. With the aim of minimizing the overuse of resources, reducing emissions associated with their production and application. For instance, AI-driven irrigation systems can significantly reduce water wastage and energy consumption, contributing to a lower carbon footprint.
2. Sustainable Crop Management
Some applications of AI in agriculture are monitoring crop health and predicting potential threats such as pests, diseases, and nutrient deficiencies. For example, drones equipped with AI-powered sensors can scan large fields, collecting data on plant health. Machine learning models can then analyze this data to identify early signs of issues, allowing for timely interventions. By preventing crop losses and reducing the need for chemical treatments, AI helps lower the emissions associated with excessive pesticide use and the transportation of goods.
3. Optimized Livestock Management
Livestock farming is a major contributor to methane emissions, a potent greenhouse gas. AI can play a crucial role in optimizing livestock management to reduce emissions. Smart sensors and AI algorithms can monitor animal health, diet, and behavior in real-time. This data-driven approach ensures that livestock are fed more efficiently, reducing the amount of feed required. Consequently, the emissions associated with feed production. Additionally, AI can help in identifying and managing waste more effectively, further reducing methane emissions.
4. Carbon Sequestration through AI-Guided Agroforestry
Agroforestry, the practice of integrating trees and shrubs into agricultural landscapes, is a proven method for carbon sequestration. AI can enhance the effectiveness of agroforestry by guiding the selection of tree species, optimizing planting patterns, and monitoring the growth of vegetation. In addition, machine learning models can predict the carbon sequestration potential of different agroforestry systems, enabling farmers to maximize their carbon capture efforts. This not only helps in reducing atmospheric CO2 levels but also enhances soil health and biodiversity.
5. Reducing Food Waste through AI-Driven Supply Chain Management
Food waste is a significant source of carbon emissions, with a large portion of waste occurring during the supply chain. For this reason, AI can help optimize supply chain logistics by predicting demand more accurately, improving inventory management, and reducing transportation emissions. For example, AI can analyze consumer behavior patterns to forecast demand, helping producers and retailers minimize overproduction and waste. Additionally, AI-driven systems can optimize delivery routes, reducing fuel consumption and associated emissions.
6. Soil Health Monitoring and Restoration
Applications of AI in agriculture can assist in monitoring soil health by analyzing data from sensors, satellite imagery, and other sources. Machine learning models can identify areas of soil degradation and recommend specific interventions, such as cover cropping or reduced tillage, to restore soil health. By improving soil carbon storage, these practices can help offset emissions and enhance the resilience of agricultural systems.
7. Predictive Analytics for Climate-Smart Agriculture
Climate change poses significant challenges to agriculture, but AI can help farmers adapt through predictive analytics. By analyzing historical climate data and current weather patterns, AI models can provide forecasts that guide planting and harvesting decisions. This allows farmers to optimize their practices in response to changing climatic conditions, reducing the risk of crop failures and minimizing the need for resource-intensive interventions. Predictive analytics also support the adoption of climate-smart agricultural practices, which contribute to lower carbon emissions.
Conclusion
The applications of AI in agriculture offers transformative potential to reduce carbon emissions and promote sustainability. From precision farming and livestock management to carbon sequestration and waste reduction, AI-driven solutions are enabling more efficient and environmentally friendly agricultural practices. As the global community continues to prioritize climate action, the adoption of AI in agriculture will play a crucial role in achieving carbon reduction goals and securing a sustainable future for the planet.
See more: Sustainable Urban Agriculture in Singapore: Latest update 2024